Op-Ed
Two A.I. Models Produced Different Results When Authenticating a Raphael Painting. Here’s Why That Doesn’t Undermine the Tool’s Potential
A.I. continues to be a reliable tool for art experts, says Carina Popovici, founder of Art Recognition.
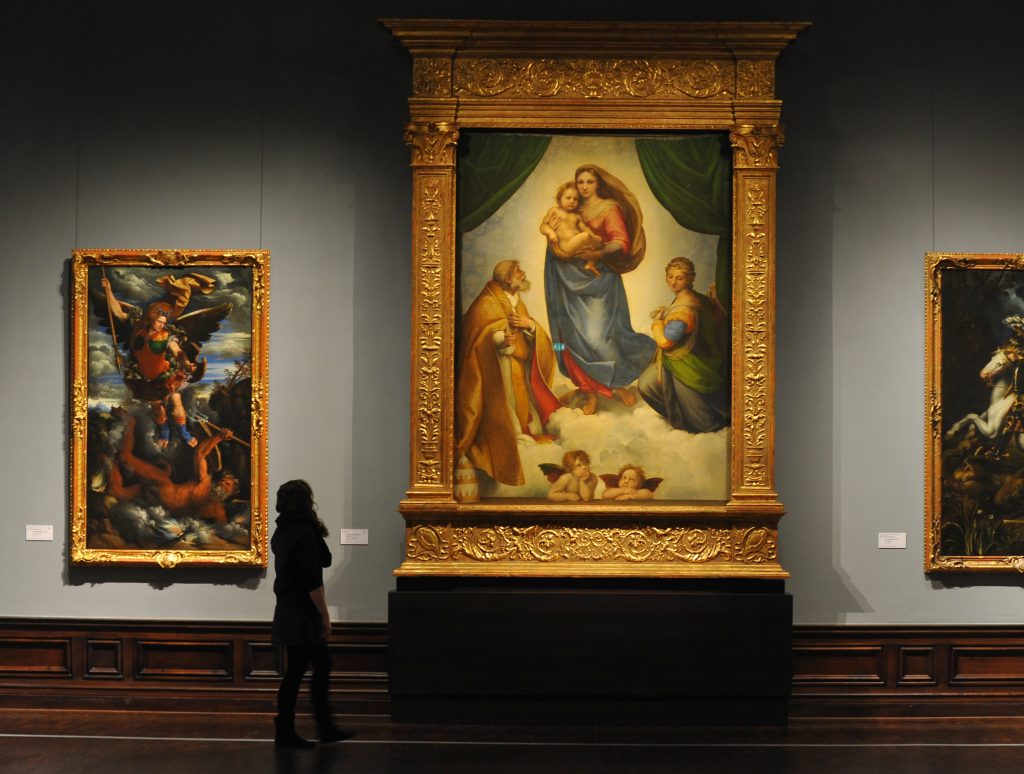
A.I. continues to be a reliable tool for art experts, says Carina Popovici, founder of Art Recognition.
by
Carina Popovici
ShareShare This Article
At the start of this year, the art world was gripped by a revelation. The painting known as the de Brecy Tondo Madonna was authenticated as a Raphael masterpiece by an A.I. system. This announcement came from a group of researchers from Bradford University in the U.K., which reported a 95 percent resemblance between the Tondo Madonna and Raphael’s Sistine Madonna, which is hanging in the Gemäldegalerie Alte Meister in Dresden.
However, this jubilation was short-lived as Art Recognition, an A.I. authentication service, made public its own A.I. analysis, which contradicted the Bradford results. According to the company, the painting was not by Raphael with an 85 percent probability.
This intriguing turn of events has sparked a fervent debate in the art world, underscoring the growing influence of A.I. in the field, and posing fundamental questions about its role and reliability in determining the authenticity of art.
To understand the situation, we must look beyond the broad “A.I.” label. The heart of the matter resides in the intricacies of A.I. authentication, since the above two systems operate on different principles.
Bradford’s artificial neural network is designed for facial recognition and is trained on datasets of faces. This facial recognition A.I. can yield a high similarity score when comparing two pictures of the same individual, regardless of the time gap between those photos, variations in the face orientation, differences in photo lightning and quality. It effectively identifies these images as depicting the same person. A logical implication of this robustness is that two paintings by different artists depicting the same face would result in a high degree of similarity.
So, how do “pictures of the same face” relate to “pictures of artworks painted by the same artist”? I put this question to lead scientist Hassan Ugail, who is one of the Bradford researchers. He acknowledged that “there is a point here,” adding that they did not conduct tests on Madonnas painted by different artists or known copies. Such tests could have shown whether the facial recognition network produced a high similarity score exclusively between the Tondo and the Dresden Madonna, rather than between the Tondo and any other Madonna paintings.
Art Recognition takes a different approach. Unlike in the model fine-tuned by the Bradford group where the effort is directed to disregard differences in the depiction of the same face, the one used by Art Recognition uses the information as to how a face has been precisely painted to elucidate the authenticity question.
The Art Recognition technology extends beyond facial recognition, encompassing broader artistic elements like brushstrokes, chromatics, and object placements. The training datasets are sourced and curated by a team of art historians and A.I. developers. They comprise photographs of validated authentic artworks by a specific artist, but also a diverse range of negative examples such as known forgeries, imitator works, and even fakes produced by a generative A.I. By exposing the A.I. model to authentic and non-authentic art, it gains the ability to distinguish genuine art from counterfeits. The result is not just a binary determination, but a probability assessment for the artwork to fall into to the “authentic” or “not-authentic” category.
For now, the most straightforward explanation for the strong discrepancy between the two results is that the models are essentially addressing different questions. The Bradford group has yet to establish a clear connection between the face similarity score and art authentication. Nevertheless, Ugail mentioned his efforts to develop a new model, and that he intends to submit his ongoing research for publication in the near future. This forthcoming scientific article will hopefully provide much-needed clarity.
Such disparities between A.I. authentication results have led to uncertainty about the process and unsure of the best course of action. On the other hand, the increasing prominence of A.I. might lead it to play a bigger role in authentication.
When it comes to A.I. authentication, several factors should be considered. First of all, the size and quality of the training datasets are critical. A robust training dataset should include a significant number of images, typically at least 100 images of authentic artworks by an artist, though this number may vary based on the artist’s complexity. A smaller dataset should raise a red flag. Secondly, rigorous testing of the model is essential. The model should undergo thorough testing on previously unseen images of artworks, both authentic and non-authentic. High accuracy on this testing set is a measure of the model’s trustworthiness.
An A.I. art authentication service can offer a convenient and cost-effective means of assessing an artwork’s authenticity. However, depending on the outcome, consulting an art expert or seeking additional scientific analyses may still be advisable. Despite the perception that A.I. poses a threat to art connoisseurs, this is far from the truth. On the contrary, A.I. is dedicated to complementing art experts and traditional authentication methods.
As A.I.’s growing influence in the art world is undeniable and irreversible, transparency and accountability become paramount. Given the complexity of A.I. models, which may be challenging for the general public to fully grasp, the scientific research underpinning A.I. art authentication should undergo rigorous scrutiny by peers and be subject to validation by the scientific community before becoming a market tool. A.I. companies must make a common effort to ensure the credibility of their products, recognizing that this endeavor benefits all stakeholders involved.
The debate is far from over, shedding light on the intricate factors that impact the process of determining the authenticity of art using A.I.. As technology evolves, so too will the discourse and the ways in which art collectors and stakeholders can effectively employ this powerful tool.
Carina Popovici is an ArtTech entrepreneur. After obtaining her PhD in physics, she worked as a quantitative risk specialist in the Swiss banking industry. Driven by her passion for art, she moved away from banking to establish Art Recognition, a startup that provides to art enthusiasts and experts an A.I. system for art authentication.