The Gray Market
Why A.I. Art Authentication Isn’t Necessarily the Game-Changer the Industry Wants It to Be (and Other Insights)
Our columnist writes up a non-techie's guide to A.I. attribution research using a freshly disputed Rubens painting.
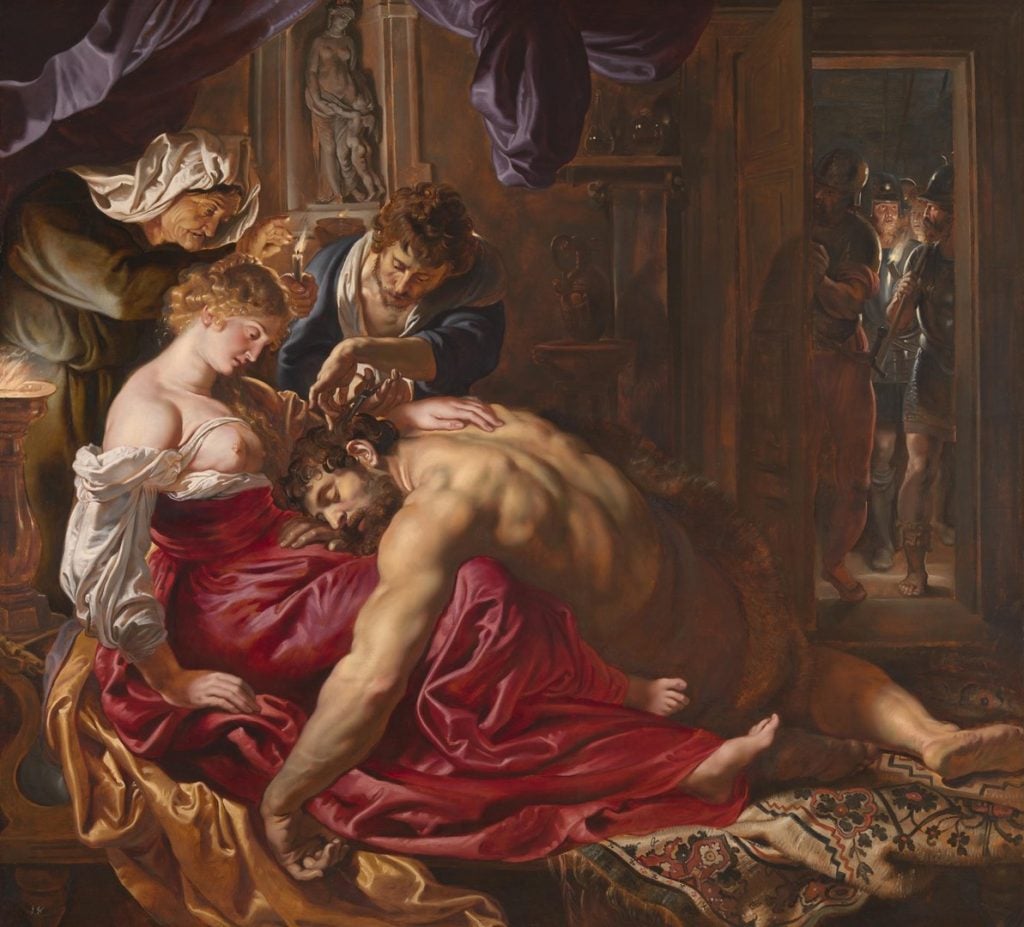
Our columnist writes up a non-techie's guide to A.I. attribution research using a freshly disputed Rubens painting.
Tim Schneider
ShareShare This Article
Every Wednesday morning, Artnet News brings you The Gray Market. The column decodes important stories from the previous week—and offers unparalleled insight into the inner workings of the art industry in the process.
This week, service journalism meets the robots…
Last month, a long-smoldering authentication dispute flared up again under the influence of new technology. Art Recognition, a Swiss firm that uses machine learning to assess the attributions of artworks, released a study whose conclusion struck like a thunderbolt: Samson and Delilah (1609–10), heretofore accepted as a Peter Paul Rubens painting in the collection of London’s National Gallery, had a 92 percent likelihood of being authored by someone other than the Old Master.
My eyebrows arched for a slightly different reason. To me, the most revealing aspect of the drama is the way it illustrates how unprepared the art world is to understand the value and limits of algorithm-backed authentication—or many other aspects of our increasingly data-driven lives.
First, the background: The National Gallery has had tremendous incentive to defend Samson and Delilah’s attribution since the day it acquired the painting at Christie’s in 1980. At the time, the institution paid a premium-inclusive £2.5 million, which the Guardian pegs at £6.6 million in the present day. (For U.S. readers, the dollar equivalents would be about $5.4 million in 1980 and $9 million today.)
That may sound like chump change in an era where a Beeple NFT racked up $69.3 million under the hammer a few months back. But 31 years ago, the National Gallery’s buy qualified as the third-highest price paid for an artwork at auction, according to the New York Times. It would also still qualify as the seventh-richest price paid for a Rubens lot after adjusting for inflation, per the Artnet Price Database. (His all-time hammer high is still The Massacre of the Innocents, 1609–11, which Sotheby’s London sold for the equivalent of $76.5 million in 2002, or more than $113 million in today’s dollars.)
But some Rubens scholars have been side-eying Samson and Delilah for decades based on traditional authentication techniques, particularly its provenance. Documents confirm Nicolaas Rockox, one of Rubens’s greatest patrons, commissioned the artist to complete a Samson and Delilah painting circa 1609. But according to research chronicled across two issues of the journal published by Old Masters advocacy group Artwatch U.K., no reliable documentation of the work’s whereabouts has been found dating to the nearly 300 years between a posthumous benefit sale of Rockox’s property in 1640, and the painting’s alleged reappearance in 1929.
The consensus among nonbelievers is that the National Gallery instead paid millions for a copy first declared an authentic Rubens by Ludwig Burchard—“an expert who, after his death in 1960, was found to have misattributed paintings by giving out certificates of authenticity for commercial gain,” per the Guardian. Skeptics have also noted points where the National Gallery’s painting deviates from the compositions of two Samson and Delilah preparatory sketches also purported to be by Rubens circa 1609 (though scholars have raised authorship questions about both of the drawings too, partly because each only emerged around the same time as the National Gallery’s painting).
No surprise, then, that these critics of the painting’s lofty attribution have been delighted by Art Recognition’s technologically advanced findings. Michael Daley, who has studied Rubens’s Samson and Delilah in his role as director of Artwatch U.K., called the study “exceedingly damning.” Scholar Katarzyna Krzyżagórska-Pisarek, who labeled the Rubens attribution “highly problematic” many moons ago, anointed algorithmic authentication “potentially groundbreaking” for the field based on Art Recognition’s results here.
The National Gallery so far is holding steady. A spokesperson said of the study, “The Gallery always takes note of new research. We await its publication in full so that any evidence can be properly assessed. Until such time, it will not be possible to comment further.”
Photo by Patrick Lux/Getty Images.
Here’s the thing: After reading both the full report and coverage of its results in multiple publications, I’m reminded that much of the art trade is missing much of the context needed to grasp what studies like Art Recognition’s do—and do not—mean.
So I’m going to swipe a page from one of my favorite podcasts, WNYC’s On the Media, by adapting its occasional Breaking News Consumer’s Handbook format. Using the Samson and Delilah dustup as a case study, here are four guidelines the average reader should keep in mind when they read or hear stories about A.I. art authentication.
(Side note: These studies actually all use machine learning, not A.I., but Silicon Valley and popular culture made up their minds to blur the distinction between the two terms long ago. Glossary here, if you need it.)
To me, the most consequential and most frequent misunderstanding about A.I. is that it is “objective.” We see the fallacy at work in the Samson and Delilah case via Krzyżagórska-Pisarek, one of the scholars skeptical of the Rubens attribution. Her praise of Art Recognition’s study included the following statement: “Devoid of human subjectivity, emotion and commercial interests, the software is coldly objective and scientifically accurate.”
This portrayal is correct in one sense—and dead wrong in another.
Experiments in data science (the broad field containing machine learning and A.I.) are just like experiments in any other branch of science: the scientists relinquish control of the results only after identifying an operative question and making numerous elemental decisions about how to design an experiment to answer it. Normally, those decisions are indeed rational, painstaking, and driven by the sole pursuit of truth, not emotions or commercial interests. But they are also still subjective, and therefore fallible.
Machine learning and A.I. are carried out through neural networks, or configurations of algorithms designed to search large amounts of data for patterns without explicit direction from humans on how to accomplish the task. And the subjective choices about neural networks start right away.
For example, what kind of neural network are you going to build to carry out your study? How many “layers” of processing nodes are needed in order to make sufficiently fine-grained distinctions in the input data? How much open-source code should you incorporate into your network architecture, and how much needs to be custom written?
Assembling the set of examples you will use to “train” your neural network to perform its task involves equally vital judgment calls. Which data should be included, and which should be omitted? What criteria do you use to decide where to draw the line? These decisions become doubly important because they also impact the size of the training dataset. (More on this soon, but for now: the more good training data you have, the more accurate and precise your neural network’s conclusions will be.)
My Opinion: Your antenna should go up anytime A.I. is celebrated as free of human influence. For a reminder of why this matters so much, think of Dr. Kate Crawford and Trevor Paglen’s ImageNet Roulette. The app showed how hundreds of thousands of pieces of actual training data pulled from a gargantuan image library used by scores of tech companies and data scientists ran rampant with embedded racial and gender biases—biases that were then learned and amplified by neural networks.
The result? Subjective, discriminatory opinions were invisibly hard-coded into allegedly “objective” A.I. tools and applied to fields like human resources and criminal justice. Neural networks aimed at authenticating artworks are just as liable to perpetuate problems if they are not designed thoughtfully. Acting otherwise gives all kinds of mistakes and mischief free rein to masquerade as innovation.
Kate Crawford and Trevor Paglen. Installation view of their exhibition “Training Humans,” at the Fondazione Prada in Milan, 2019–2020. Photo by Marco Cappelletti, courtesy Fondazione Prada.
Research takes all shapes and sizes. On one end of the spectrum, you have peer-reviewed academic papers that stretch to reams of pages, thanks in part to the inclusion of detailed methodologies and full reproductions of the raw data collected. On the other, you have independent analyses spanning only a few pages, often conducted by a single researcher with no supervision, a minimally descriptive methodology, and little to none of the raw data included. A vast gulf separates the two poles.
This isn’t to say that conclusions made by small studies created with limited resources are uniformly wrong. But the less transparency is provided, and the less oversight is involved, the more questions you should have about the findings. This goes double for studies carried out by neural networks because machine learning itself is a black box; even the data scientists involved often struggle to pinpoint how, exactly, a neural network is evaluating the input data it’s fed.
In this case, Art Recognition’s entire Samson and Delilah report consists of six pages: three of which are a title page, a glossary of data science terms, and a page containing an image of the painting with its basic details and the one-line summary judgment, “The Art Recognition A.I. System evaluates Samson and Delilah NOT to be an original artwork by Peter Paul Rubens with a probability of 91.78 percent.”
At the same time, Art Recognition’s cofounders have serious academic and business credentials, according to the company’s website. Dr. Carina Popovici holds a PhD in theoretical particle physics and worked in risk management in Switzerland’s banking industry; Christiane Hoppe-Oehl has an advanced degree in mathematics and spent 20 years in the Swiss banking sector, primarily building financial forecasting models.
The company also boasts a three-person advisory board: professor Eric Postma of Tillburg University in the Netherlands; art dealer and Sotheby’s Zurich veteran Ully Wille; and Jason Bailey, the journalist, collector, and art-tech entrepreneur behind Artnome. The Samson and Delilah report does not mention whether, or how, these advisors were involved.
My Opinion: Art Recognition’s team is experienced, but despite a reproduction of the “heat map” showing which areas of the painting mattered most to the neural network’s verdict, its study is still short and relatively cagey, especially in comparison to peer-reviewed academic research. (I reached out to the founders over the weekend, and Popovici offered a few more answers in response to my questions, which are incorporated throughout.)
Update, October 13: After publication, Popovici stated, “The report which we have prepared for Samson and Delilah is not written for A.I. specialists, and is by no means a summary of our research; rather, it is meant to give some insight into our work to the non-technical reader. Currently we are finishing a scientific publication together with our academic partners from Tilburg University and will submit it to a peer-reviewed journal for publication.”
Peter Paul Rubens, The Raising of the Cross (ca. 1610/11). Collection of Onze Lieve Vrouwe-Kerk, Antwerp.
Art Recognition’s Samson and Delilah conclusion is informed by analysis of “high quality” photographic reproductions of 148 paintings “fully created by Rubens (and not finished by his workshop),” according to the company’s report. Also included in the training data is an unspecified number of contrast images known to be made by other artists with similar styles from a similar era.
This process purports to unearth the “main features” that define Rubens’s unique artistic style. The idea is that Art Recognition’s convolutional neural network—a specific type of machine-learning structure attuned to evaluating 2D images—can more clearly see what makes a Rubens a Rubens by also being exposed to what is not a Rubens (but also not radically different).
Although the report later references “other structural characteristics… not related to the artistic representation,” the only specific features mentioned are brushstroke patterns. These, again, are being analyzed from photographs or scans that, the report notes, vary in quality.
My Opinion: I’ll give Art Recognition the benefit of the doubt on the overall high quality of the Rubens images (and thus, brushstroke reproductions) used in its training data. Brushstroke patterns seem like a useful trait in identifying a single artist’s aesthetic fingerprint, too. After all, traditional authenticators have been examining them for decades.
However, Art Recognition’s report offers no indication of who or what is being relied upon to label the 148 Rubens paintings in its training data as “fully created by” the artist. I mention this because plenty of respectable scholarship on Rubens argues his assistants were so consistently skilled that it is frequently difficult, if not impossible, to parse where their contributions end and the master’s begin. Rubens was even known to outsource preparatory sketches to some of them. In the recent Rubens: A Genius at Work, the late art historian Arnout Balis posits—in a chapter literally titled “Rubens and His Studio: Defining the Problem”—that “it remains for Rubens studies in the future to delineate just how diverse the the possibilities of execution were for works ‘by Rubens’ (that is, works produced in his name and for which he took responsibility.)”
With so many complications around attributing authorship throughout Rubens’s career, it becomes all the more important to specify what criteria were used to decide the 148 allegedly unassisted Rubens paintings in Art Recognition’s training data form the basis of his unique brushstroke signature. Again, it’s a judgment call. Combine this omission with the mystery surrounding the other “structural characteristics” the firm’s neural network is assessing, however, and the results feel wobbly.
Art Recognition’s Samson and Delilah report describes its “final, balanced” training dataset as containing “2,392 data [points] stemming from original [Rubens] images and a similar number of data points in the contrast set.”
Wondering how the firm got to this magnitude of total data points from 148 uncontested Rubens paintings and the aforementioned contrast images by Rubens’s artist peers? To dial in on fine details, Art Recognition subdivided each Rubens painting into smaller, non-overlapping “patches” whose sizes “depend on the image quality and the size of the brushstrokes.” The patches were then cropped into squares, rearranged, and evaluated individually.
So the neural network derived info on the macro structure of Rubens paintings by evaluating high quality photographic reproductions of the full originals, and info on the micro-structures of Rubens paintings by evaluating the patches and sub-components. Art Recognition also adjusted select “hyperparameters,” or factors that influence the speed and manner in which the algorithm learns, to optimize accuracy.
This approach may sound rigorous and robust to people new to data science and machine learning, but it did not impress any of the people with deep experience in those fields who I spoke to.
Addie Wagenknecht, Sext (2019). Courtesy of the artist.
One member of that group is Addie Wagenknecht, who has worked with neural networks as an artist and tech professional for years. She described any machine-learning study based on the datasets described in Art Recognition’s report as “comical.” The bare minimum training set she could trust, she estimated, would consist of 10,000 data points, or about double the amount the study indicates was used here (i.e. 2,392 Rubens data points, plus a “similar number” in the contrast images). Otherwise, she would need the firm to validate its neural network by training it on an equally small dataset of works by a living artist who could personally verify the results.
When I told Popovici about the pushback I was getting on the size of the training datasets for authenticating Old Masters, she said she agreed that “data scarcity is a problem. There are, however, different data augmentation techniques available to increase the number of data points in the training set, some of which we are also using.”
It’s unclear to me whether those data augmentation techniques extend beyond the use of contrast images and patch analysis described in the Samson and Delilah report. Popovici did not provide further details about Art Recognition’s methodology, saying only, “This is a long discussion and I’m tired.” She did not respond to my offer to talk the next day.
My Opinion: Since the consensus I’m hearing from more experienced people is that the Samson and Delilah dataset is small enough to raise eyebrows, I’m uncomfortable too.
It’s true that small sample sizes can sometimes still reveal legitimate trends. It’s just that they are also much more liable to accidentally illustrate anomalies than are larger datasets. For instance, I can think of three- or four-day stretches of springlike weather in Brooklyn last winter, but the average temperature during those stretches was still a blissful outlier compared to the frigid season as a whole.
I’m not saying Art Recognition’s Samson and Delilah report captures the artistic equivalent of those few unseasonably warm days—just that experts I trust say we need more data to know one way or the other.
Update, October 13: After publication, Popovici said that the total number of data points in the training set was 4,750: the combination of 2,392 data points in the Rubens images deemed original, plus 2,358 data points in the set of contrast images. (These two categories—original Rubens works, and works by other artists—make up the two “classes” of data assessed by Art Recognition’s neural network.) Descriptions of the dataset above have been adjusted to account for this clarification.
Popovici stated, “The number of classes we deal with in a classification problem is crucial in order to correctly assess the size of the training dataset. The general consensus in the specific literature is that a good dataset should contain 1,000 to 2,000 data points per class. Therefore, depending on the number of classes, the size of the training dataset can vary from 5,000 to millions of data points. Many deep learning models are trained on higher numbers of classes (like separating email categories, just to give a random example), and consequently a larger training dataset is required. But that doesn’t mean there is something wrong with training on only two classes. The size of our dataset is just fine.”
Popovici also stated, “Standard data augmentation techniques (other than splitting the images into patches) are flipping, mirroring, rotation etc. We found that these types of artefacts don’t have an influence on the results. That is why I did not insist on them.”
The National Gallery’s faith in the attribution of Samson and Delilah to Rubens seems questionable to me, but that’s because of the traditional case against its provenance and compositional anomalies, not the algorithmic one. Art Recognition’s report makes an interesting side note, and it could be meaningful with a more robust dataset and more transparency about its methodology. As it stands, though, it’s a long way away from being the smoking gun the press is making it out to be.
I think it’s also a cautionary tale about every future A.I.-driven authentication story to come, particularly concerning works by Old Masters. Most artists in the genre have relatively few extant works in pristine condition with sterling provenance, and even the examples beyond reproach are often known to be executed by varying levels of input from assistants, each with their own unique tendencies. All of these aspects undermine the foundation that neural networks must stand on to deliver trustworthy results.
But that won’t stop data scientists, authenticators, and art-market actors from trotting out would-be bombshells dropped by A.I. Instead, prepare for a future where different neural networks backed by different groups deliver conflicting judgments about the likely authorship of artworks with imperfect credentials, especially when financial stakes stretch into the millions of dollars. (I shivered thinking about what happens if Salvator Mundi, whose much-disputed attribution to Leonardo was also backed by the National Gallery, returns to the market.) But at least when the floodgates open, you’ll have a handbook to help you navigate the murky waters.
That’s all for this week. ‘Til next time, remember: What you leave out is just as important as what you put in.