Analysis
Has Artificial Intelligence Brought Us the Next Great Art Movement? Here Are 9 Pioneering Artists Who Are Exploring AI’s Creative Potential
There's a lot going on in the world of art and AI.
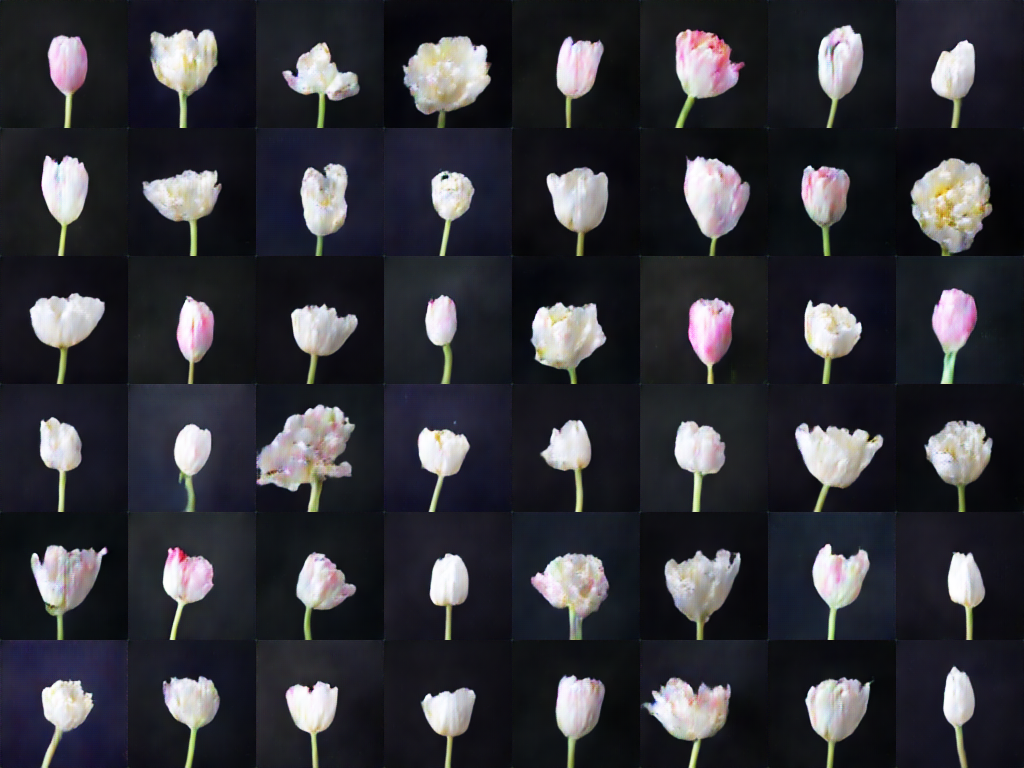
There's a lot going on in the world of art and AI.
Naomi Rea
ShareShare This Article
Art-market history was made late last month when a work created using a neural network—one type of technology now commonly, if somewhat reductively, classified as an artificial intelligence—sold at Christie’s auction house in New York for $432,500.
The print was made using a form of machine learning called a Generative Adversarial Network, or a GAN (which you can read more about here). The Christie’s sale wasn’t the first time a work using so-called AI has been sold at auction, but it did mark the first time that a major auction house paid attention to the field—and helped drive the price up to nearly half a million dollars.
The sale has also stirred controversy in the close-knit generative-art community. Many artists working in the field point out that Obvious, the collective behind the AI that made the pricey work, was handsomely rewarded for an idea that was neither very original nor very interesting. The savvy marketers behind the sale seemingly took advantage of the fact that the wider public is not very educated about the sector. Even the Christie’s expert who put the sale together admitted that he first learned about Obvious’s work from an article that appeared on artnet News in April.
As for the other major auction houses, a spokesman for Bonhams, interestingly, told us it is not considering hopping on the algorithmic trend now or in the future. Neither Sotheby’s nor Phillips responded to requests for comment on the matter.
While we wait for the next AI-generated work to hit the block, there’s a lot more to learn. To find out about the interesting work being created with machine learning—and the complex boundaries it’s pushing—we’ve assembled a list of nine pioneering artists to watch.
An image of Albert Barqué-Duran carrying out the live painting inspired by the muse generated by Klingemann’s algorithm. Image courtesy the artists.
WHO: Klingemann is an artist whose preferred tools, rather than a paintbrush and paints, are neural networks, code, and algorithms. He taught himself how to program in the ’80s, so you could see his algorithms as Outsider AI-artists. (Just kidding!) He’s currently an artist-in-residence at Google Arts and Culture (which brought us that viral art historical face-matching app). He also runs a gallery space in Munich called Dog & Pony, and his works have been shown at the Ars Electronica Festival, the Museum of Modern Art in New York, the Metropolitan Museum of Art, London’s Photographers Gallery, and the Centre Pompidou, among others.
WHAT: Klingemann works with generative models (i.e. GANs) a lot, which he sees as “creative collaborators.” Recently, he collaborated with one of these neural network partners as well as a more traditional artist, Albert Barqué-Duran, on a project titled My Artificial Muse. The project sought to create a computer-generated “muse” to inspire a painting. For this, Klingemann trained his network on a database of more than 200,000 photographs of human poses until it was able to generate new ones. Then, based on the information it was fed about poses and paintings, the network generated an image, which was scaled up and then painted in mural form during a live performance by Barqué-Duran.
WHY: In exploring machine learning, Klingemann hopes to understand, question, and subvert the inner workings of systems of all kinds. He has a particularly deep interest in human perception and aesthetic theory, subjects he hopes to illuminate through his work creating algorithms that show almost autonomous creative behavior.
Tulips from Mosaic Virus (2018). Image courtesy the artist.
WHO: Ridler has a more traditional artistic background than some of the others on this list. She has degrees from the Royal College of Art, Oxford University, and University of the Arts London. Although her work essentially uses the same code architecture as Klingemann’s, she generates her own data sets to train her models rather than using existing images. She says this gives her more “creative control” over the end result. Creating bespoke data sets is demanding: it consumes around 60 to 70 percent of the total time it takes her to create a work. She’s shown her work at Ars Electronica, Tate Modern, and the V&A, among other institutions.
WHAT: Ridler usually uses GANs, as she believes they produce some of the most visually interesting results. For her current project, Mosaic Virus, she’s using something called spectral normalization (a new technique that helps the algorithm generate better-quality images). She created a training set by taking 10,000 photos of tulips over the course of tulip season and categorizing them by hand. Then, she used the software to generate a video showing her tulips blooming—but their appearance was controlled by the fluctuations in the price of bitcoin, with the stripes on the petals reflecting the value of the cryptocurrency. The work draws historical parallels between the “tulip mania” that swept Europe in the 1630s (when prices for tulips with striped petals caused by the mosaic virus soared) and the current speculation on cryptocurrencies. For other works, such as The Fall of the House of Usher, she trained her neural network on her own ink drawings.
WHY: “The most interesting part of working with machine learning is the way that it repeats your idea back to you, but in a way that is looser and wilder and freer than I could ever make by myself,” Ridler tells artnet News. “Looking at the work that I’ve made with AI is always like catching a glimpse of yourself in a mirror before you recognize that it is you—you but also not you.”
Some outfits the Balenciaga AI made that are vastly different than what already exists;
• A jumpsuit type piece of clothing (even covering feet)- paired with a coat.
• What looks like a small pants-included bag that you fold onto your shin?
• An interestingly patterned top pic.twitter.com/yxmIYoNsdm
— Robbie Barrat (@DrBeef_) August 22, 2018
WHO: Barrat is a 19-year-old artist and coder who currently works in a research lab at Stanford University. He is notable not only for his youth, but also for the humor and originality of his creations. When he was in high school, he created this rapping neural network trained on Kanye West’s entire discography. Back in 2017, he also uploaded the code for a very similar project to the one Obvious just cashed in on at Christie’s for anyone to play around with (which is looking increasingly suspect for Obvious). Another of his models, interestingly, misinterpreted the data set of nude portraits that it was fed, giving too much attention to things like rolls of flesh and bellybuttons to generate a series of trippy, Francis Bacon-esque nudes.
WHAT: For a recent project, Barrat has been working at the intersection of fashion and machine learning. Using Balenciaga’s catalogues as training data, he is having a series of neural networks generate new outfits, and even a brand new runway show. So far, he tells artnet News, “the outfits that the machine makes are a lot more strange and more asymmetric than anything Balenciaga has put out.” He and a designer collaborator Mushbuh are currently working with a factory in Pakistan to make some of the algorithm-generated designs a reality. I don’t know about you, but I can definitely see the shin-bag becoming a thing.
WHY: Like other artists working with machine learning, Barrat sees AI as a tool or medium. He views it as a collaboration between artist and machine.
The latest print by Tom White, Mustard Dream (2018). Courtesy the artist.
WHO: Tom White is a New Zealand-based artist who is currently working as a lecturer in computational design at the University of Wellington. He specializes in “creative coding” and was included in the first mainstream gallery show dedicated to art made using artificial intelligence, which we wrote about earlier this summer.
WHAT: White works with neural networks called Convolutional Neural Networks, or CNNs. These networks are used in today’s computer vision applications to give modern machine-learning systems the ability to perceive the world through vision—for example, systems that filter obscene images from your Google search. In his work, White investigates the perceptual abilities of these systems by finding abstract forms that are meaningful to them. Trained on a set of images of real-life objects, the machine creates abstract representational prints until the forms created register as the specific objects, such as a starfish or a cabbage, when they are run through other AI systems to confirm. Some of the results register as “very likely obscene” when they are run through systems trained to filter obscene content, even though they might not register that way to us humans. See the above “obscene” print by White for reference.
WHY: White says working with artificial intelligence is like coming up against a staunchly different culture with completely foreign ways of seeing. In his own words, “By using AI techniques to generate my prints, I both learn and communicate how these systems differ in what they understand visually. This gives some insight in how the intelligences we are creating often agree but sometimes differ in their understanding of the world we share.”
Helena Sarin, Pretty in GAN courtesy the artist.
WHO: Sarin is a traditional artist who uses GAN variants to transform and enhance her own pencil-on-paper sketches. She has been building commercial software for a long time, but only recently, with the discovery of GANs, has she been able to align her twin passions of coding and art.
WHAT: Sarin pretty much exclusively uses something called CycleGAN, a GAN variant that does image-to-image translation. She essentially trains a network to transform images with the form of one data set to have the textures of another data set. For example, she translates her photos of food and drink into the style of her still-lifes and sketches of flowers. She explains that one of the perks of using CycleGAN is that she can work in high resolution, even with small data sets. Plus, the model trains relatively quickly.
WHY: “As a software engineer and an artist, I always wanted to combine these two tracks of my life,” Sarin explains. “But the generative art in processing was too abstract for my taste. On the other hand, the generative models trained on my art keep producing organic folksy imagery that almost never fails to excite and surprise me.”
Gene Kogan, Style Collage—Jackson Pollock (2018). Courtesy the artist.
WHO: Kogan is an artist and programmer interested in how generative systems, computer science, and software can be used creatively for self-expression. He collaborates on several open-source software projects and lectures about the intersections of code and art. He’s also literally written the book on machine learning for artists.
WHAT: Like many of the people on this list, Kogan trains neural networks, a popular type of machine-learning software, on images, audio, and text. He says his primary goal is to develop generative models, or to help teach the software to output fresh, varied work based on inputs. He is particularly interested in cross-pollinating media—teaching the neural networks to output music based on a drawing of an instrument, for example.
WHY: “It lets me try to find out interesting things about the collective mind or ‘hive mind,’ a bank of knowledge composed of all of our data,” Kogan explains of AI’s appeal. “I am interested in the collective imagination, what we reveal about ourselves by our data in aggregate. I am also interested in using neural networks to create new forms of interaction which can respond to rich sensory data, like vision, sound, and natural language.”
Samim Winiger, from DeepGagosian (2018). Courtesy Samim Winiger.
WHO: Winiger is a self-described “designer and code magician” who used to produce dance music for the likes of Diplo and Shaggy. The Berlin-based developer, who doesn’t define himself as an artist per se, is known for his experiments with artistic computational creativity experiments.
WHAT: Winiger says his network of choice “very much depends on the occasion.” (They range from Convolutional Neural Nets (CNNs) to Recurrent Neural Nets (RNNs) to Dimensionality Reduction techniques (TSNE etc.) and beyond, but we won’t get into that here.) Recently, he’s been using neural networks to explore “DeepFakes,” living hoaxes created by software taught to, say, swap one person’s head onto another’s body in one or more images. Once it has been trained on enough examples for the task at hand, a machine can produce an entire video hoax without the need for frame-by-frame manipulation on the programmer’s part. (In fact, since some DeepFake software now uses graphical interfaces—think: clicking and dragging icons—rather than coding, almost anyone can do this now.) Just for artnet News, and for fun, he trained a custom neural network he calls “DeepGagosian,” which places the face of the famous art dealer Larry Gagosian in different scenes. He’s used this to insert Mr. Gagosian into a few unusual contexts, such as a drug gang bust and a gathering of heads of state (see above).
WHY: Asked to pinpoint the most interesting part of working with neural networks for creative purposes, Winiger says: “When using machines to assist with the creation of creative artifacts, questions of authorship and agency are very much blurred. By using augmentation tools, the human creator is (in the best case) freed up to focus on the core message of the piece.” And with generative toolboxes like DeepFakes becoming more readily available, gone are the days when you needed mad Photoshop skills to pull off a convincing faked image (like the ones in DeepGagosian). Winiger explains: “We are currently at a major inflection point, where the cost of high quality image manipulation has dropped to close to 0. This concludes a 100+-year relationship with photography, where the common ‘photography equals reality’ assumption is breaking down.”
Sofia Crespo, {External_Anatomy 1020}. Courtesy the artist.
WHO: Crespo is a Berlin-based artist and art director. Her previous work includes Trauma Doll, an algorithm-powered doll that “suffers” from PTSD, anxiety, depression, and other mental health issues. As a coping mechanism, the doll creates collages using pattern recognition from online texts including memes, philosophical texts, and news headlines.
WHAT: Crespo is currently working on a project called Neural Zoo, which explores how creativity combines known elements in a specific way in order to create something entirely new. In her view, the creator in this case would be the algorithm itself, but with a human artist as its muse. “Isn’t all art made by humans an execution or reshaping of data absorbed through biological neurons?” Crespo asks. “How can we continue to inspire machines to create art for us as emotional human beings?”
WHY: “An interesting thing this experience gave me is the change of perspective in the design role,” Crespo says. “I originally went from designing a layout and features for an image, to actually designing the data and letting a program decide those features by itself. I guess my role became trying to anticipate what the model could create with the given data, and it never stops surprising me.”
Sougwen Chung, Drawing Operations (2017). Courtesy the artist.
WHO: Chung is a Canadian-born, Chinese-raised, New York-based interdisciplinary artist and former research fellow at MIT’s Media Lab. She’s currently E.A.T.’s artist-in-residence in partnership with the New Museum and Bell Labs. Her work, which spans installation, sculpture, drawing, and performance, explores mark-making by both hand and machine in order to better understand the interactions between humans and computers. She has exhibited at institutions including The Drawing Center in New York and the National Art Center in Tokyo.
WHAT: For her current project, Drawing Operations, Chung uses Google’s TensorFlow, an open-source software library used for machine learning, to classify archives of her own drawings. The software then transfers what it has learned about Chung’s style and approach to a robotic arm that draws alongside her. She’s also working on a few new projects using pix2pix (a neural network trained to produce variations on an image, like the nighttime version of a daytime photo) and sketch-rnn (which tries to continue or complete a digital sketch based on where the human leaves off) to expand on this idea of human and machine collaboration.
WHY: “As an artist working with these tools, the promise of AI offers a new way of seeing,” Chung explains. “Seeing as self reflection, seeing through the ground truth of ones own artworks as data. There is a lot of talk about biases evident in AI systems and that is absolutely true within AI systems trained on art. You could describe visual language as a kind of visual bias, a foregrounding of the subjective view of the artist. By translating that into machine behavior, I am attempting to create a shared intersubjectivity between human and machine.”