Art World
How Did A.I. Art Evolve? Here’s a 5,000-Year Timeline
Artists have been employing artificial intelligence from ancient Inca knots to modern-day GANs.
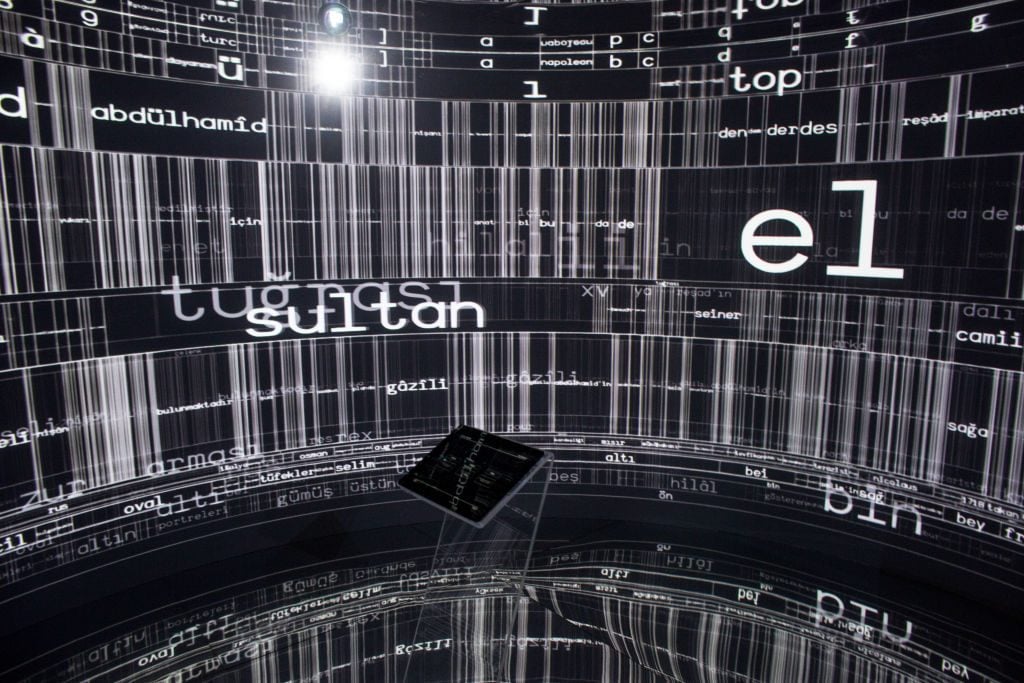
Artists have been employing artificial intelligence from ancient Inca knots to modern-day GANs.
Naomi Rea
ShareShare This Article
The term “artificial intelligence” has been colored by decades of science fiction, where machines capable of thinking freely, learning autonomously, and maybe even experiencing emotions have been reimagined in different forms, whether that has been as benevolent as WALL-E or as malevolent as HAL-9000. So it is perhaps not our fault that when we hear about A.I. art, we might picture something that is actually a major misconception of the technology.
The oracular entity we imagine as the maestro behind such artworks is what researchers today would call an “artificial general intelligence,” and while technologists are actively working toward this, it does not yet exist. “I think a lot of people like to ascribe somewhat spiritual qualities to A.I. as it is something beyond human ken, something that is more pure in that way,” A.I. artist and researcher Amelia Winger-Bearskin said. “But it is actually quite messy—it’s just a bunch of nerdy coders and artists that are making stuff.”
While the fiction of A.I. art is pretty neat, the messy reality is that artists who work with computational systems have much more say in the outcomes than the term might suggest: they provide the inputs, guide the process, and filter the outputs. Artists have been attracted to using A.I. in their work for a variety of reasons; some are drawn to working with the most futuristic technologies, others use it as a way of integrating chance into their work, and others see potential for it to expand elements of their existing practices.
Below we’ve outlined a timeline of a few of the key developments within the long history of A.I. art.
Model of a Jacquard loom (Scale 1:2), 1867. Photo by Science Museum/SSPL/Getty Images.
A.I. didn’t spring forth from nothing in the 21st century. Here are its earliest seeds.
3000 B.C. – Talking Knots
The ancient Inca used a system called Quipu—”talking knots”—to collect data and keep records on everything from census information to military organization. The practice, in use centuries before algebra was born, was both aesthetically intricate and internally logically robust enough that it could be seen as a precursor to computer programming languages.
1842 – Poetical Science
Ada Lovelace, often cited as the mother of computer science, was helping researcher Charles Babbage publish the first algorithm to be carried out on his “Analytical Engine,” the first general purpose mechanical computer, when she wrote about the idea of “poetical science,” imagining a machine that could have applications beyond calculation—could computers be used to make art?
The functionality of the analytical engine was actually inspired by the system of the Jacquard loom, which revolutionized the textiles industry around 1800 by taking in punch card instructions for whether to stitch or not—essentially a binary system. A portrait of the loom’s inventor Joseph Jacquard, woven into a tapestry on the loom in 1836 using 24,000 punched cards, could in this sense be viewed as the first digitized image.
1929 – A Machine That Could See
Austrian engineer Gustav Tauschek patented the first optical character recognition device called a “reading machine.” It marked an important step in the advance of computers, and prompted conversations familiar to those evoked by artificial intelligence today: What does it mean to look through machine eyes? What does a computer “see?”
1950 – The Imitation Game
Alan Turing developed the Turing Test, also known as Imitation Game, a benchmark test for a machine’s ability to exhibit intelligent behavior indishtinguishable from a human.
Art works of Jean Tinguely are seen prior to the ‘Jean Tinguely. Super Meta Maxi’ exhibition at Museum Kunstpalast on April 21, 2016 in Duesseldorf, Germany. Photo by Sascha Steinbach/Getty Images.
1953 – Reactive Machines
Cybernetician Gordon Pasks developed his “MusiColour” machine, a reactive machine that responded to sound input from a human performer to drive an array of lights. Around the same time, others were also developing autonomous robots that responded to their environments, such as Grey Walter’s Machina Speculatrix Tortoises Elmer and Elsie, and Ross Ashby’s adaptive machine, Homeostat.
1968 – Cybernetic Serendipity
Artists in the 1960s were influenced by these “cybernetic” creations, and many created “artificial life” artworks that behaved according to biological analogies, or began to look at systems themselves as artworks. Many examples were included in the 1968 “Cybernetic Serendipity” exhibition at London’s Institute of Contemporary Art. Bruce Lacey exhibited a light-sensitive owl, Nam June Paik showed his Robot K-456, and Jean Tinguely provided two of his “painting machines,” kinetic sculptures where visitors would get to choose the color and position of a pen and the length of time the robotic machine operated, and it would create a freshly drawn abstract artwork.
1973 – An Autonomous Picture Machine
In 1973, artist Harold Cohen developed algorithms that allowed a computer to draw with the irregularity of freehand drawing. Called Aaron, it is one of the earliest examples of a properly autonomous picture creator—rather than creating random abstractions of predecessors, Aaron was programmed to paint specific objects, and Cohen found that some of his instructions generated forms he had not imagined before; that he had set up commands that allowed the machine to make something like artistic decisions.
Although Aaron was limited to creating in the one style Cohen had coded it with—his own painting style, which lay within the tradition of color field abstraction—it was capable of producing an infinite supply of images in that style. Cohen and Aaron showed at Documenta 6 in Kassel in 1977, and the following year exhibited at the Stedelijk Museum in Amsterdam.
By the late 20th century, the field began to develop more quickly amid the boom of the personal computer, which allowed people who did not necessarily come from a tech background to play with software and programming.
By the time the 2000s rolled around, the field opened up considerably thanks to resources specifically geared toward helping artists learn how to code, such as artist Casey Reas and Ben Fry’s Processing language, and open-source projects accessible on the Github repository. Meanwhile, researchers were creating and making public vast sets of data, such as ImageNet, that could be used to train algorithms to catalogue photographs and identify objects. Finally, ready-made computer vision programs like Google DeepDream allowed artists and the public to experiment with visual representations of how computers understand specific images.
Amid all these innovations, developments in the field of AI art began branching and overlapping. Here are three main categories.
The landing page for Lynn Hershman Leeson’s Agent Ruby (2010). Courtesy the artist.
While these software applications are omnipresent fixtures in lieu of live customer service agents, some of the earliest iterations were used by artists.
1995 – A.L.I.C.E
Richard Wallace’s famous A.L.I.C.E. chatbot, which learned how to speak from gathering natural language sample data from the web, was released in 1995.
2001 – Agent Ruby
Artist Lynn Hershman Leeson was working nearly concurrently with Wallace on her own chatbot as part of an artistic project commissioned by SFMOMA in 1998. Leeson had made a film called Teknolust, which involved a cyborg character with a lonely hearts column on the internet who would reach out and talk to people. Leeson wanted to create Agent Ruby in real life, and worked with 18 programmers from around the world to do so. Agent Ruby was released in 2001, and Leeson said she did not really see it as a standalone A.I. artwork at the time but more as a piece of “expanded cinema.”
2020s – Expanded Art
Since then, many artists have created works involving chatbots. Martine Rothblatt’s Bina48 chatbot is modeled after the personality of her wife, and Martine Syms has made an interactive chatbot to stand in for her digital avatar, Mythiccbeing, a “black, upwardly mobile, violent, solipsistic, sociopathic, gender-neutral femme.”
In this photo illustration a virtual friend is seen on the screen of an iPhone on April 30, 2020, in Arlington, Virginia. Photo by Olivier Douliery/AFP via Getty Images.
There are many ways in which artists are working with A.I. to create generative art, using various kinds of neural networks—the interconnected layers of processing nodes, modeled loosely on the human brain—as well as machine learning techniques such as evolutionary computation. But by far the most commonly associated with A.I. art today are Generative Adversarial Networks—or GANs.
2014 – GANs are developed
Researcher Ian Goodfellow coined the term in a 2014 essay theorizing that GANs could be the next step in the evolution of neural networks because, rather than working on pre-existing images like Google DeepDream, they could be used to produce completely new images.
Without getting too technical, there are two things to understand about how a GAN works. First, the “generative” part: the programmer trains the algorithm on a specific dataset, such as pictures of flowers, until it has a large enough sample to reliably recognize “flower.” Then, based on what it has learned about flowers, they instruct it to “generate” a completely new image of a flower.
The second part of the process is the “adversarial” part—these new images are presented to another algorithm that has been trained to distinguish between images produced by humans and those produced by machines (a Turing-like test for artworks) until the discriminator is fooled.
Anna Ridler, Tulips from Mosaic Virus (2018). Image courtesy the artist.
2017 –The Birth of GANism
After Goodfellow’s essay about GANs was published in 2014, tech companies open-sourced their raw and untrained GANs, including Google (TensorFlow), Meta (Torch), and the Dutch NPO radio broadcaster (pix2pix). While there were a few early adopters, it took until around 2017 for artists to really begin to experiment with the technology.
Some of the most interesting work has been made when the artists don’t look at the algorithm as completely autonomous, but use it to independently determine just some features of the work. Artists have trained generative algorithms in particular visual styles, and shaped how the model develops creatively by curating and honing the outputs to their own tastes, meaning they can vary widely in aesthetic and conceptual depth. Some train the algorithms on datasets of their own work—like Helena Sarin, who feeds in her drawings, or Anna Ridler who uses her own photographs—and others have scraped from public data to ask conceptually interesting questions—such as Memo Akten, who for his 2018 film Deep Meditations trained a model on visually diverse images scraped from Flickr that were tagged with abstract concepts relating to the meaning of life—allowing the machine to offer up its own eerie interpretation of what our subjective collective consciousness suggests these things have in common.
2018 – Auction Milestone
Probably the most famous example of a GAN-made artwork in the contemporary art world is a portrait made by the French collective Obvious, which sold at Christie’s in 2018 for a whopping $432,000. The trio of artists trained the algorithm on 15,000 portraits from the 14th to 20th century, and then asked it generate its own portrait, which in a stroke of marketing genius, they attributed to the model.
The resulting artwork—Portrait de Edmond de Belamy (the name an homage to Goodfellow)—which vaguely resembled a Francis Bacon, captured the market’s attention. While there has been much debate about the aesthetic and conceptual importance of this particular work, the astronomical price achieved counts it as an important milestone in the history of A.I. art.
Obvious Art’s ??? ? ??? ? ?? [??? ? (?))] + ?? [???(? − ?(?(?)))], Portrait of Edmond de Belamy, Generative Adversarial Network print on canvas (2018).
In recent years, there has been an increasingly large cohort of artists who are looking at A.I. not necessarily to produce images, but as part of a practice addressing how A.I. systems and inherent algorithmic biases impact issues of social justice, equity, and inclusion.
2019 – ImageNet Roulette Goes Viral
While there are many artists working on these questions, a standout moment came when artist Trevor Paglan and researcher Kate Crawford’s ImageNet Roulette project went viral.
Their project aimed to expose the systemic biases that humans have passed onto machines by looking at the specific case of the ImageNet database, a free repository of some 14 million images that were manually labelled by tens of thousands of people as part of a Stanford University project to “map out the entire world of objects.” The database is widely used by researchers to train A.I. systems to better understand the world, but because the images were labelled by humans, many of the labels are subjective, and reflect the biases and politics of the individuals who created them.
Paglan and Crawford’s project allowed the public to upload their own image to the system for it to label what it understood to be in the image. The database classified people in a huge range of types including race, nationality, profession, economic status, behavior, character, and even morality. And plenty of racist slurs and misogynistic terms came within those classifications. Scrolling through Twitter at the time, I remember seeing people sharing their own labels: a dark-skinned man is labeled as “wrongdoer, offender;” an Asian woman as a “Jihadist.”
It was a poignant illustration of a hugely problematic dimension of these systems. As Paglen and Crawford explained: “Understanding the politics within AI systems matters more than ever, as they are quickly moving into the architecture of social institutions: deciding whom to interview for a job, which students are paying attention in class, which suspects to arrest, and much else.”
Exhibition view of “Kate Crawford, Trevor Paglen: Training Humans” Osservatorio Fondazione Prada, through Februrary 24, 2020. Photo by Marco Cappelletti, courtesy Fondazione Prada.
2020s – A Generation of Activist A.I. Art
Other artists working in this vein include some of those early pioneers of A.I. art such as Lynn Hershman Leeson, whose interactive installation Shadow Stalker (2018–21) uses algorithms, performance, and projections to draw attention to the inherent biases in private systems like predictive policing, which are increasingly used by law enforcement.
Elsewhere, artists like Mimi Onuoha have focused on “missing datasets” to highlight bias within algorithms by thinking about all the types of representations of data that we don’t have, and has created a series of libraries of these datasets, such as the missing data focused on Blackness. Meanwhile, artists like Caroline Sinders have activist projects like the ongoing Feminist Data Set, which interrogates the processes that lead to machine learning—asking of each step in the pipeline, from data collection to labeling to training, is it feminist? Is it intersectional? Does it have bias? And how could that bias be removed? Or Joy Buolamwini, who uncovered flaws in facial recognition technology struggling to identify faces with darker skin tones, and who interrogates the limitations of A.I. through artistic expressions informed by algorithmic bias research.