Analysis
A.I. Art Valuation Is the Market’s Holy Grail. Here Are 7 Reasons Why It’s Harder Than It Sounds
From data bias to information opacity, there are a lot of reasons why it's going to take a while for A.I. to predict the value of your art.
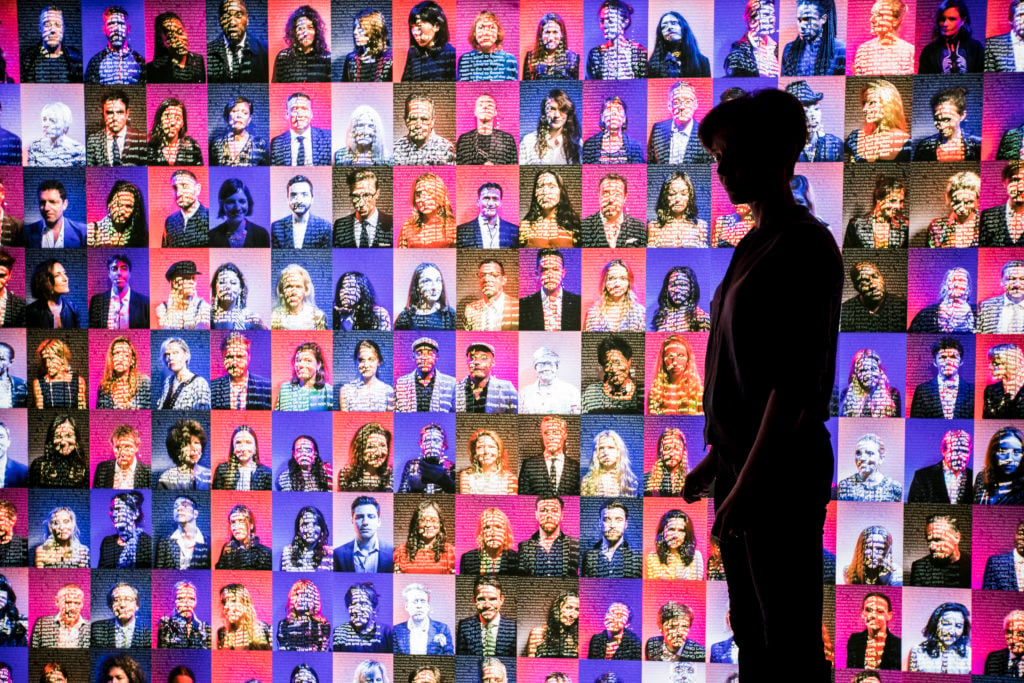
From data bias to information opacity, there are a lot of reasons why it's going to take a while for A.I. to predict the value of your art.
Tim Schneider
ShareShare This Article
A version of this story first appeared in the spring 2020 Artnet Intelligence Report and is part of our cover package on Artificial Intelligence. For more, read our thorough breakdown of how A.I. could change the art business and our glossary of A.I. terminology.Â
Despite how eager dealers, collectors, and advisors are for trustworthy algorithmic art-pricing predictions, several core obstacles currently stand in the way—all courtesy of perhaps the quirkiest market known to humankind.
So before you buy into any A.I. company that claims to predict the value of art, beware of the following complications.
Â
To bring real value, any A.I. application needs loads of quality data—which is doubly problematic in our small and notoriously opaque industry (dealers l-o-v-e to hoard sales info). Without greater transparency, A.I. can do only so much.
Â
As the old computing adage goes: garbage in, garbage out. Any art-market algorithm fed data spoiled by past prejudice—say, decades spent overvaluing Western, white male painters—will produce equally rotten conclusions unless proper controls are programmed in.
Â
Few unique artworks are created equal, even those within the same body of work by a single artist. This fact adds noise to all sales data based on comparisons to similar artworks, making predictive valuation thorny.
Â
The essence of deep machine learning is that even the developers don’t know exactly how or why the algorithms reach their conclusions about data sets. This leaves open the possibility that the under-lying mechanisms could be flawed in ways that outsiders can’t identify—until the A.I.’s seemingly infallible predictions suddenly go haywire.
Â
Even the best predictive models are vulnerable to chance—just ask Wall Street. From global economic crises to whether a promising artist will be derailed by a drug problem, not everything that matters to the market can be accounted for.
Â
No matter what the data tells you, an artwork is worth whatever someone can be convinced to pay for it. Just because a sleek algorithm forecasts a juicy price for a painting doesn’t necessarily mean a like-minded buyer will materialize on the same time horizon.
Â
Any effective A.I. application centralizes a massive amount of data in-house, giving the developer the option to license the product for others’ use…or simply guard its secrets to capture all the value for itself.